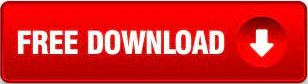

- #RAISE AMOUNT OF BINS OF HISTOGRAM IN EXCEL 2016 FOR MAC#
- #RAISE AMOUNT OF BINS OF HISTOGRAM IN EXCEL 2016 INSTALL#
- #RAISE AMOUNT OF BINS OF HISTOGRAM IN EXCEL 2016 UPDATE#
- #RAISE AMOUNT OF BINS OF HISTOGRAM IN EXCEL 2016 MANUAL#
- #RAISE AMOUNT OF BINS OF HISTOGRAM IN EXCEL 2016 DOWNLOAD#
Activating “Analysis Tool Pack” in Excel Macįollow these simple steps to activate “Analysis Tool Pack” in Excel Mac
#RAISE AMOUNT OF BINS OF HISTOGRAM IN EXCEL 2016 DOWNLOAD#
…make sure to download this sample file to follow along.
#RAISE AMOUNT OF BINS OF HISTOGRAM IN EXCEL 2016 INSTALL#
To create a histogram in the Mac version of Excel we need to install “Analysis Tool Pack” as well.
#RAISE AMOUNT OF BINS OF HISTOGRAM IN EXCEL 2016 FOR MAC#
#RAISE AMOUNT OF BINS OF HISTOGRAM IN EXCEL 2016 UPDATE#
When you add a new value in the main data it will not update it, so you need to create a new chart.
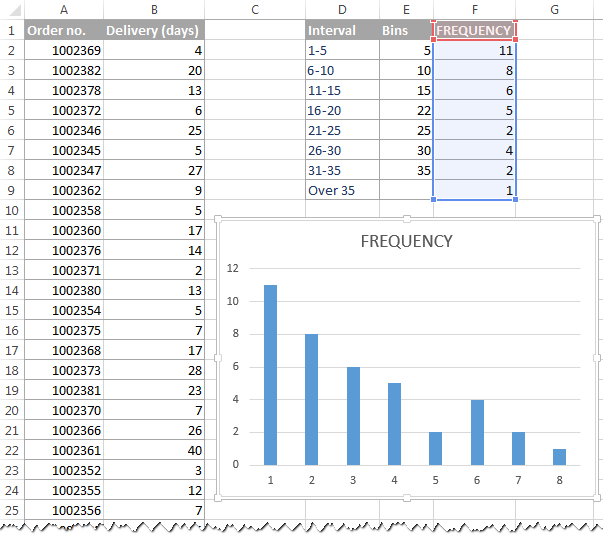
#RAISE AMOUNT OF BINS OF HISTOGRAM IN EXCEL 2016 MANUAL#
Numpy's manual page on histogram_bin_edges provides nice list and pros and cons of each approach. Then, one should find a stable/robust method that does not change with changing data. If binning changes the inference or results of the upstream task. Experience tells, this depends on the upstream task as well. With so few data, what approaches should I take to calculating the number of bins to use?įD or doane methods (see below) might be more suitable. Spike histograms are similar to rug plots, and the human eye is quite good at summarizing distributional shapes from examining tick mark density in the rug. You'll also see an example where horizontal lines are added underneath the histogram to show various quantiles as in a box blot. Many examples are shown here including interactive spike histograms where data values can be viewed in hover text. The key algorithm is here in for example the histboxp function. The result is "spike histograms" which I've implemented in many functions in the R Hmisc package. For that reason I use either m=100 or 200 bins regardless of the sample size, with modifications to (1) have unequally spaced bins when the number of distinct data values is not huge and (2) to pool such unequally spaced bins when two distinct data values are closer together than, say, 1/5m of the data span. I believe that histograms need to be both summary and descriptive measures. This clashes with the need to show individual outliers, digit preference, bimodality, data gaps, and other features. Scroll past that to see the theory and explanation, then keep scrolling to find links to the papers that explain the method.Ĭonventional wisdom dictates that a "broken look' resulting from a histogram with many bins is undesirable. It is a bit more complicated to calculate, but seems to do a great job.
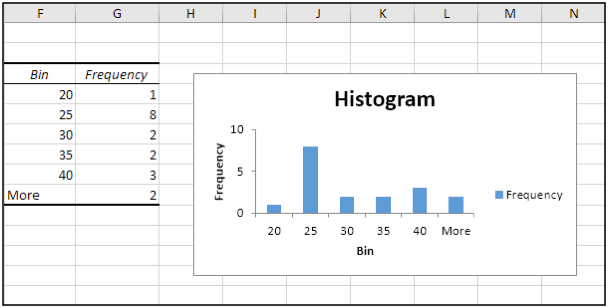
This page from Hideaki Shimazaki explains an alternative method. The simplest method is to set the number of bins equal to the square root of the number of values you are binning. This wikipedia page lists several methods for deciding bin width from the number of observations. If you have lots of values, your graph will look better and be more informative if you have lots of bins. The decision clearly depends on the number of values.
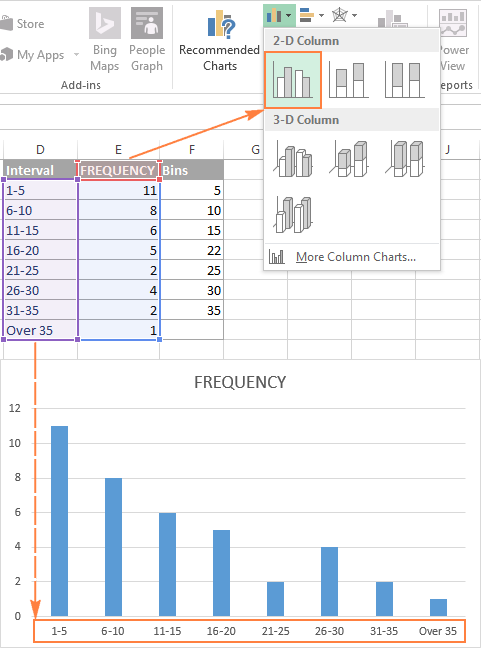
If you want to create a frequency distribution with equally spaced bins, you need to decide how many bins (or the width of each). Either a dot plot, or a cumulative frequency distribution, which doesn't require any bins. One solution is to create a graph that shows every value. If you have too many bins, you get a broken comb look, which also doesn't give a sense of the distribution. If you use too few bins, the histogram doesn't really portray the data very well.
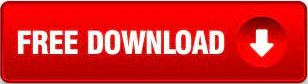